In December, OpenAI announced a new model, o3, which represented a significant advancement toward AGI. But what does this new release mean in terms of the numbers and some of the predictions that drive our insights at FPOV?
OpenAI recently ran their o3 model on a benchmark for AGI called ARC-AGI, and scored exceptionally well, reaching a score of 87.5% in one configuration of the model. To provide some context, GPT-3 scored 0%, and GPT-4o only scored a 5%! So, there’s been huge strides in reasoning and generalized problem-solving capabilities even since 4o. A testament to the power of the new architecture of the reasoning models, compared to the GPT series.
OpenAI ran o3 on this test in a couple of configurations, essentially low and high compute, with the high compute being the version headlining at 87.5% of AGI. What’s the difference? The high compute configuration used 172x the compute resources as the low-compute version.
But how much compute is that?
This graphic does a great job at portraying the key differences:
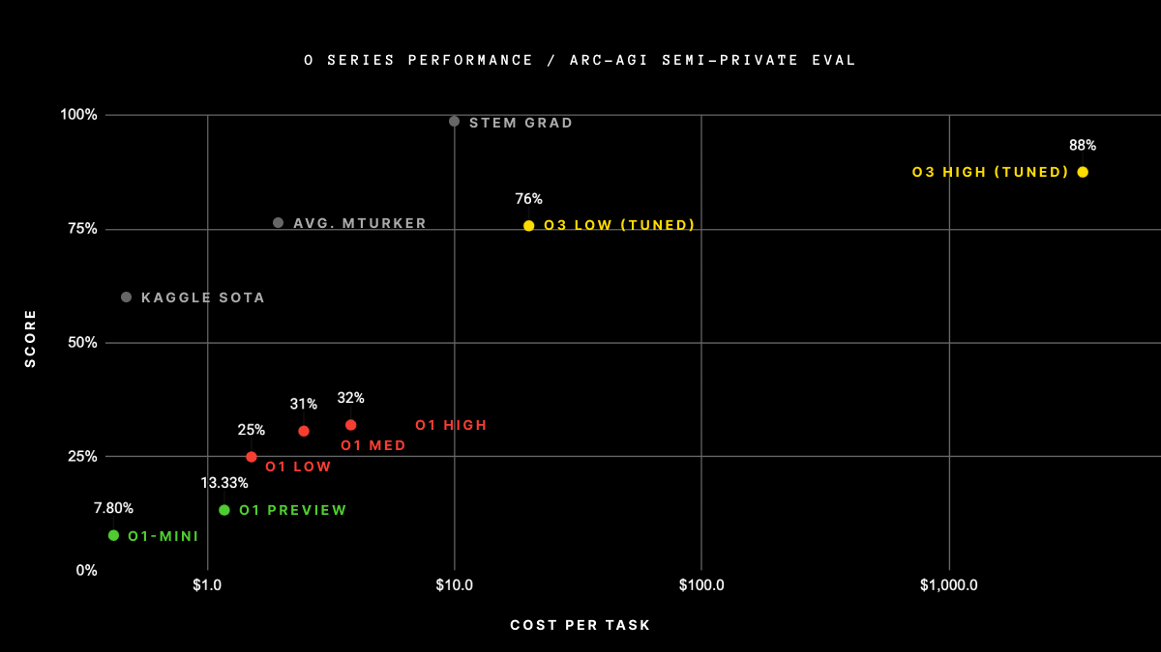
Signup for our Newsletter
The ARC-AGI’s results show OpenAI omitted the computational cost for the high-compute version, however, we were able to determine a good estimate to the real numbers.
They ran two tests which contained 100 and 400 tasks, respectively. I found that the retail costs for the 100 tasks were $390k and the 400 task version was around $1.15MM…. just to perform the tests. This works out to about
2,871−
3,490 per task!
The cost of AI-related compute costs has been rising significantly, especially driven by the release of Large Reasoning Models (LRMs).
Enter DeepSeek…
DeepSeek is a Chinese artificial intelligence (AI) company that has recently garnered significant attention for its advancements in AI model development. On January 20, 2025, DeepSeek released its latest AI model, DeepSeek-R1, designed to enhance complex problem-solving capabilities. This model has been made fully open-source under the MIT license, allowing for free use and modification by researchers and developers
The introduction of DeepSeek-R1 has led to significant market reactions, particularly among U.S. technology stocks. DeepSeek’s V3 model was trained in approximately 55 days at a cost of around $5.58 million, utilizing significantly fewer resources compared to its peers (Wikipedia).
Companies heavily invested in AI infrastructure, such as Nvidia, Microsoft, and Alphabet, experienced notable stock declines in reaction to the release. This market response reflects investor concerns about the potential for more cost-effective AI models to disrupt existing business models and valuations.
DeepSeek also appears to signal a disruption in compute costs. DeepSeek’s models are designed for efficient inference. For instance, the Reasoner model operates as a cost of \$0.55 per million tokens processed, which is substantially lower than OpenAI’s o1 model, which charges \$15 for the same number of tokens. (Business Insider)
DeepSeek’s breakthrough has many investors questioning the viability of the incumbent frontier models and the massive investments they’ve taken for training and inference.
However, many are pointing to the winds of AI shifting in favor of inference. As more models reach relative parity with OpenAI (Gemini, DeepSeek, etc) the race will shift away from model training to model inference – the actual use (compute) of the foundation LLMs.
Model commoditization and cheaper inference could lead to more widespread adoption, meaning that the investment in the industry will be viable to meet the increased demand. This theory is supported by the Jevons Paradox, which describes a counterintuitive phenomenon where technological progress that increases the efficiency of resource use leads to increased consumption of that resource, rather than decreased use.
But it is worth noting that while DeepSeek currently offers drastically cheaper inference than ChatGPT, models like Gemini 1.5 Flash are actually cheaper, and DeepSeek is set to increase its token costs in February.
What does this mean for organizations?
In the world of emerging technology, disruptions can come at any time and present challenges for existing processes and workflows. Especially in the realm of artificial intelligence, it will be crucial for organizations to build dynamic AI strategies that mitigate for disruption by avoiding over-reliance on any single provider.
About the Authors
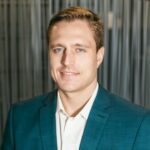
Trent Saunders
Trent’s natural curiosity for emerging technology makes him a great addition to FPOV’s Business Development team. As Business Expansion Manager, Trent leverages his passion for pitching new concepts to evangelize the FPOV offerings. Learn more about Trent Saunders.
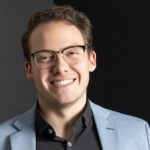
Riley Howell
Riley Howell is a multi-faceted leader with deep engineering and technical experience. He possesses proficiency in both mechanical systems design and software development, with a track record of successful implementation. Riley is a proven project leader and thrives providing unique solutions to complex problems. Learn more about Riley Howell.